AI in Pharma | The future of drug discovery and development
MedSocial, San Jose, California, October 14, 2019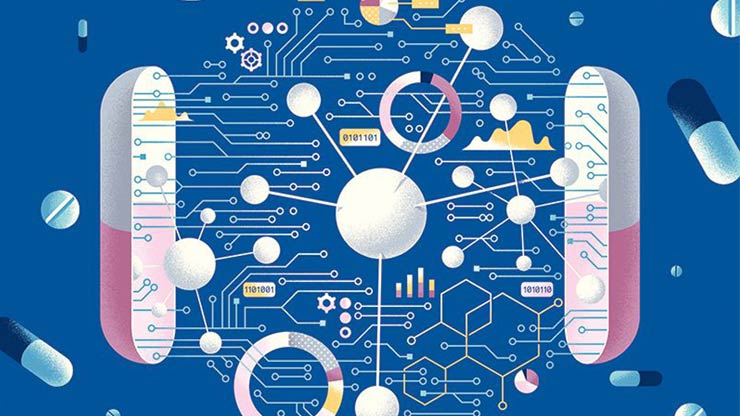
To say, ‘artificial intelligence (AI)’ has become a buzzword in business would be an understatement. Although this term was coined way back in 1956, it gained much prominence recently after it was considered as a potential threat to replace humans in future jobs. However, the recent spate of investments in the AI-driven drug discovery indicate the interest that this segment is attracting in the pharmaceutical and biotech industries. In fact, companies like Merck, Roche, Bayer and Pfizer are already partnering with emerging AI-driven startups and even acquiring some of them to boost their own drug discovery processes.
Roadblocks to drug discoverys
The drug discovery process is typically arduous, long, and expensive. An average drug takes 12 years to reach the market and costs around 2.6 billion US Dollars. Such R&D investments are spread over several years and with a mere 13.8% success rate , these often put a massive burden on the organization. Hence, according to Forbes , the more the pharmaceutical industry improves the standard of care, the more difficult and costly it becomes for it to improve the standard of care further.
One way to resolve this dilemma is to outsource drug research to academic institutions and private contract research organizations. According to a report by Visiongain , drug discovery outsourcing business was estimated to be at $19.2 billion in 2016. Reducing the R&D cost, increasing the pace of drug discovery and accessing specialized knowledge and technologies are some reasons that are encouraging pharma companies to outsource drug research to third parties.
Another emerging solution is to utilize the growing capabilities of artificial intelligence for drug discovery and development. With rapid advances in AI, pharma companies can realize faster drug discovery and reduce costs. A PWC report titled ‘Why AI And Robotics Will Define New Health’ states, “By directing the latest advances in AI to streamline the drug discovery and drug repurposing processes, there is the potential to significantly cut both the time to market for new drugs and their costs, not only for the labs who develop drugs, but for those people whose health depends upon them.”
However, the lack of expertise about this nascent technology limits the use of AI in drug discovery. A survey of 330 drug discovery researchers revealed that more than 40% of scientists were unfamiliar with AI’s capabilities in drug discovery. The study also highlighted that 62% of the participants believed that the lack of knowledge was the most significant barrier that prevented them from adopting AI for drug discovery.
Current use of AI in drug discovery
The process of drug discovery involves testing chemical compounds against diverse cell types, different genetic mutations, varied side effects and numerous other factors. Since there are often millions of chemical compounds that may initially meet these criteria, scientists are often limited as to how many compounds they can potentially investigate and how many can be sent for clinical trials, which inherently limits the number of compounds that can be studied and increases R&D costs substantially.
Fortunately, AI-based algorithms allow computers to be “trained” on the previously processed data and predict future outcomes. For example, researchers at the University of California trained such algorithms to scan more than 3 million commercially available compounds and found a new class of painkillers.
When the Zika virus broke out in 2015, AI made a significant contribution by helping scientists in finding a treatment. In this new Nobel Prize-winning process, instead of growing crystals, scientists used a nearly frozen solution of the disease protein. This solution contained lots of protein particles that represented the disease protein in random orientations. The best particles from the solution, identified by an algorithm, were then photographed using an electron microscope to generate terabytes of images at different orientations. Using AI, these images were then analyzed to create a detailed three-dimensional model of the disease protein, thereby shortening the discovery process of finding the drug from years to four weeks.
A drug design company, Atomwise, is using deep convolutional neural networks to facilitate structure-based drug design. This company uses its AI-based platform called AtomNet to screen and analyze ten million small molecules daily so as to predict their potency related to medications, toxicity, and side effects. This approach helps in avoiding the manual process of tweaking and over-parameterizing the binding features and helping it to successfully predict new active molecules as potential targets (with no previously known modulators).
Another British drug design company, Exscientia, uses AI to discover drugs for complex diseases, where the target mechanisms are often unknown. Exscientia’s phenotypic design platform utilizes big data analysis to extract high-dimensional phenotypic readouts of the key performance markers. These readouts help in the generation and optimization of new iterations of drug compounds. Given this backdrop, it is not surprising that the market for AI-propelled drug discovery is set to be a $4 billion market by 2024.
Large companies getting in the fray
Companies like Bayer, AstraZeneca, GSK have already partnered with other AI-driven drug research companies to strengthen their R&D, and some have even directly invested in them or acquired them. For example, GSK invested $300 million in 23andMe , a Silicon Valley gene testing company that has more than five million customers; this gives GSK access a vast DNA database, providing information about the relations between genes and diseases.
Similarly, in 2018, Roche acquired Flatiron Health for $1.9 billion. Flatiron Health is a market leader in oncology-specific electronic health record software as well as in the development of real-world evidence for cancer research. Flatiron Health’s large network of community oncology practices and academic medical centers across the US along with its technology platform, can allow both companies to accelerate progress towards data-driven personalized medicine.
Pfizer is using IBM Watson to search for immuno-oncology drugs. IBM Watson uses medical information of patients to optimize the decision making by studying millions of research papers.
Year | Pharmaceutical Company | AIbased Company | Technology |
---|---|---|---|
December 2018 | Merck | Cyclica | AI-driven cloud based in-silico proteome screening platform |
September 2018 | Pfizer | Atomwise Inc. | Using AI for drug discovery |
July 2018 | GSK | 23andMe | Access to DNA database |
July 2018 | Eli Lilly | Transcriptic | AI-driven on-demand drug discovery |
April 2018 | Roche | Flatiron Health | Oncology-specific electronic health record software |
February 2018 | Bristol-Myers Squibb | Sirenas | Machine-learning computing to discover therapeutics |
May 2018 | Boehringer Ingelheim | Bactevo | Totally Integrated Medicines Engine for drug discovery |
September 2017 | Sanofi | Exscientia | Discover bispecific small molecules for diabetes |
June 2017 | Genentech | GNS Healthcare | Find potential cancer drug targets |
Sources: BiopharmaTrend & BenchSci
Way ahead
Many pharmaceutical and biotech companies are already leveraging AI for drug target identification and validation, disease identification and diagnosis, drug discovery, drug repurposing, clinical trial research, and poly-pharmacology discovery. In addition, AI is also being used for managing data, conducting repetitive tasks, analyzing healthcare systems, medication management, and medical consultations.
The vast potential of AI in drug discovery will also eventually lead to personalized medicine, and with its help, treatment could be designed for each patient with a specific biological profile. Companies like Deep Genomics are already using advanced AI platforms to interpret genetic variations, which could help understand the connections between genetics, proteomics and specific diseases.
Finally, in the coming years, the influence of AI in pharma will grow far beyond drug discovery. Already, there are AI-based social platforms like MedSocial that use advanced text processing at large scale to decipher and analyze millions of online messages from across multiple sites and geographical locations. This platform understands unstructured data and extracts relevant topics, sentiments, and trends for a drug and provides competitive analysis and deep insights.